Notice
Recent Posts
Recent Comments
Link
일 | 월 | 화 | 수 | 목 | 금 | 토 |
---|---|---|---|---|---|---|
1 | 2 | |||||
3 | 4 | 5 | 6 | 7 | 8 | 9 |
10 | 11 | 12 | 13 | 14 | 15 | 16 |
17 | 18 | 19 | 20 | 21 | 22 | 23 |
24 | 25 | 26 | 27 | 28 | 29 | 30 |
Tags
- sbert
- Enriching Word Vectors with Subword Information
- 동적토픽모델링
- ROC-AUC Curve
- 분류모델평가
- word2vec
- word representation
- type-hint
- 허깅페이스
- 구글클라우드플랫폼
- topic modeling
- 알파베타가지치기
- Holdout
- GCP
- 의미연결망
- Google Cloud Platform
- semantic network
- 토픽모델링
- degree centrality
- 머신러닝
- 사회연결망분석
- QANet
- 감성분석
- sensibleness
- 임베딩
- sequential data
- Min-Max 알고리즘
- Meena
- dynamic topic modeling
- hugging face
Archives
- Today
- Total
목록OOV (1)
Dev.log
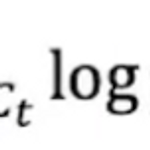
본 포스팅에서는 FastText(Enriching Word Vectors with Subword Information)논문에 대해 리뷰해 보도록 하겠습니다. FastText는 2017년 ACL에서 발표되었으며, 오늘날 워드임베딩의 개념인 word vectors representation을 학습시키는 방법에 대한 논문입니다. 본 논문이 등장 할 때 까지만 해도 워드 임베딩(word embedding)이라는 단어 대신 distributed 혹은 continious word represnetation이라는 단어가 주로 사용되었습니다. FastText은 Word2vec을 타겟 논문으로 잡았으며, word2vec의 몇가지 한계점에 대해 언급했습니다. Word2Vec의 한계점 Word2Vec의 한계는 하나의 단어에..
논문리뷰
2022. 3. 4. 10:41